Complete Guide to Principal Component Analysis
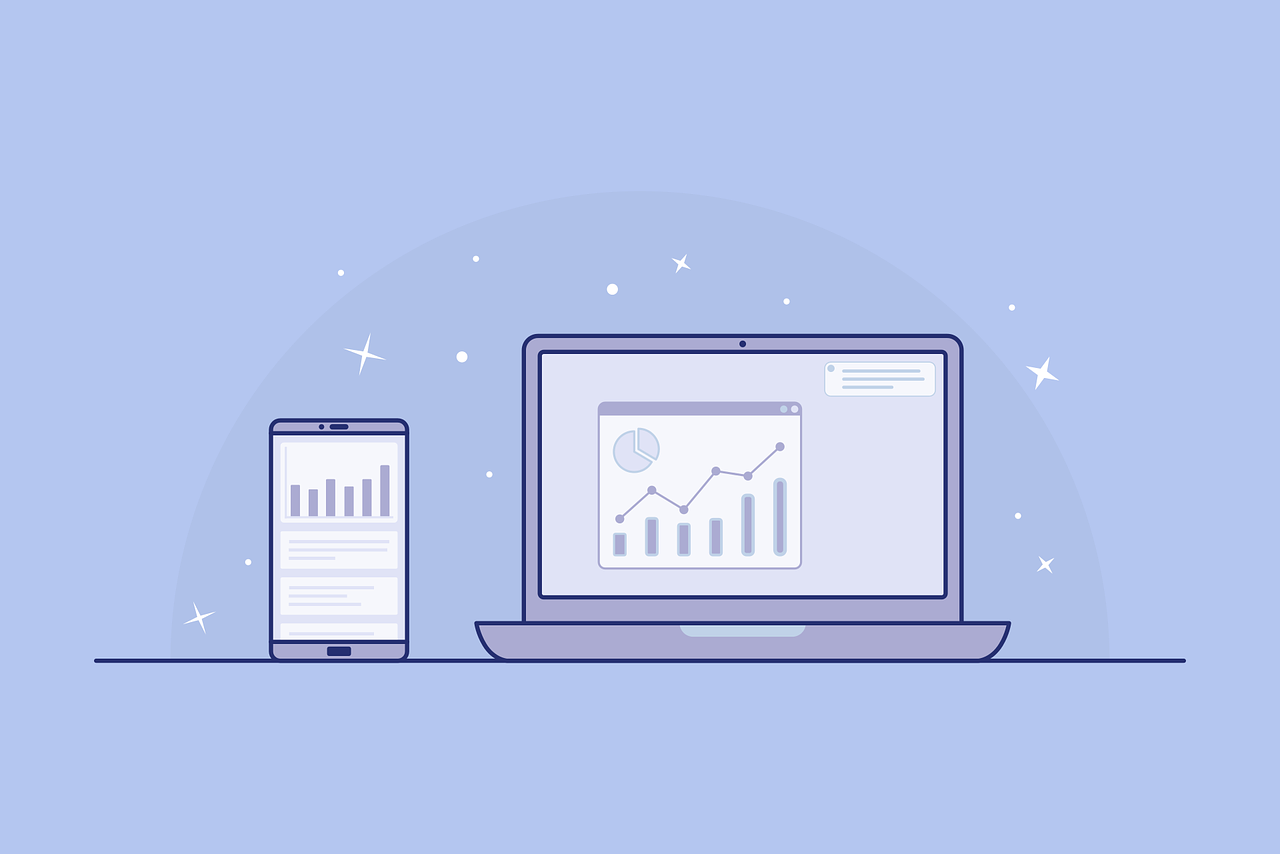
PCA vs. Factor Analysis
PCA and factor analysis are both dimensionality reduction techniques. However, they have different objectives. While PCA aims to find the most significant components that explain the data variance, factor analysis aims to uncover latent factors or constructs that underlie the observed variables. Additionally, factor analysis allows for the possibility of measurement error, which is not considered in PCA.
Conclusion
Principal Component Analysis is a powerful tool for dimensionality reduction and data exploration. By identifying the most significant patterns in high-dimensional datasets, PCA simplifies complex data analysis tasks. Understanding the underlying mathematics and interpreting the results are crucial for applying PCA effectively. Despite its limitations, PCA remains a valuable technique in various domains and continues to contribute to the advancement of data analysis and machine learning.