Complete Guide to Principal Component Analysis
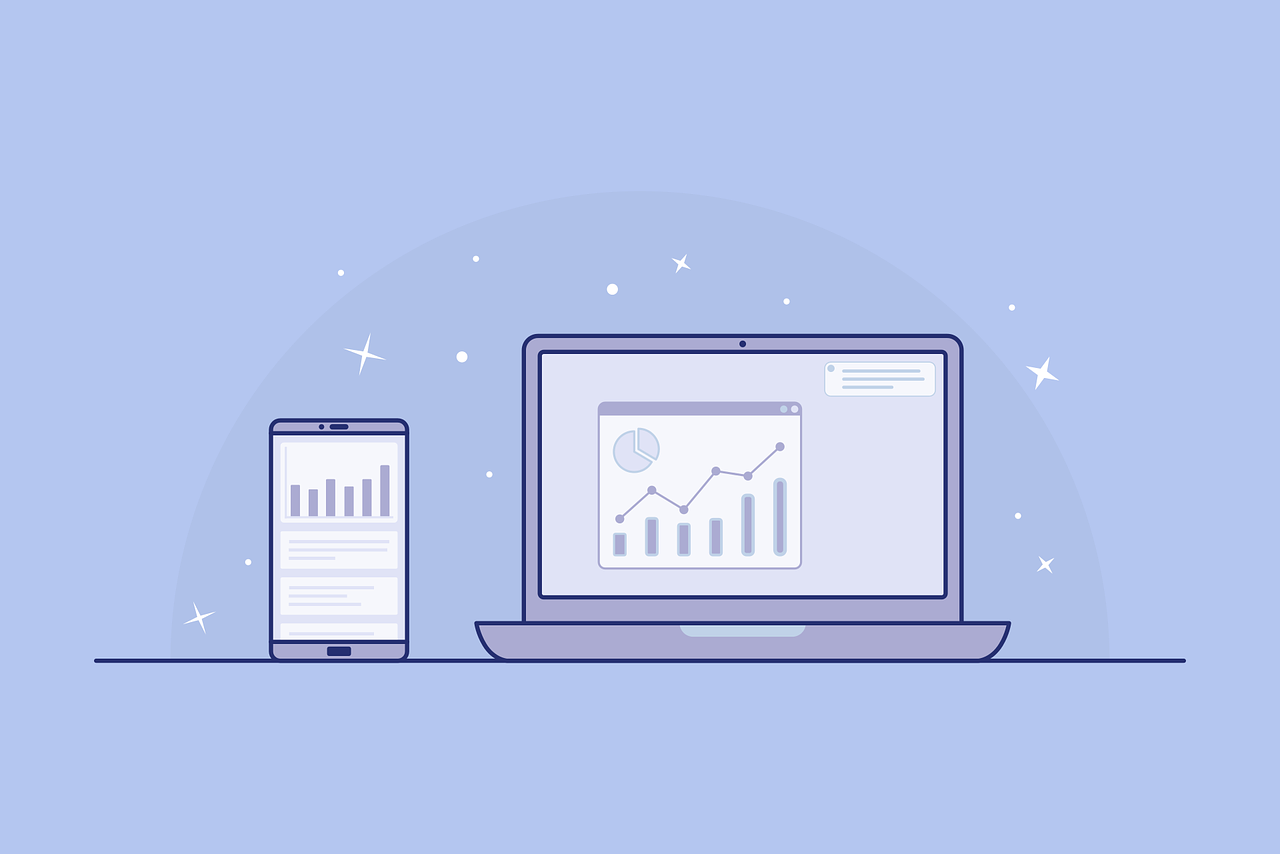
FAQ 1: What is the purpose of Principal Component Analysis?
Principal Component Analysis (PCA) aims to reduce the dimensionality of a dataset while preserving the most important information. By transforming the data into principal components, PCA helps in visualizing and understanding complex datasets.
FAQ 2: How do eigenvalues and eigenvectors relate to PCA?
Eigenvalues and eigenvectors play a crucial role in PCA. Eigenvalues represent the variance or importance of a particular component, while eigenvectors define the direction or pattern associated with it. Combining eigenvalues and eigenvectors allows us to evaluate the importance and interpretation of the principal components.
FAQ 3: How do I choose the number of principal components in PCA?