Complete Guide to Principal Component Analysis
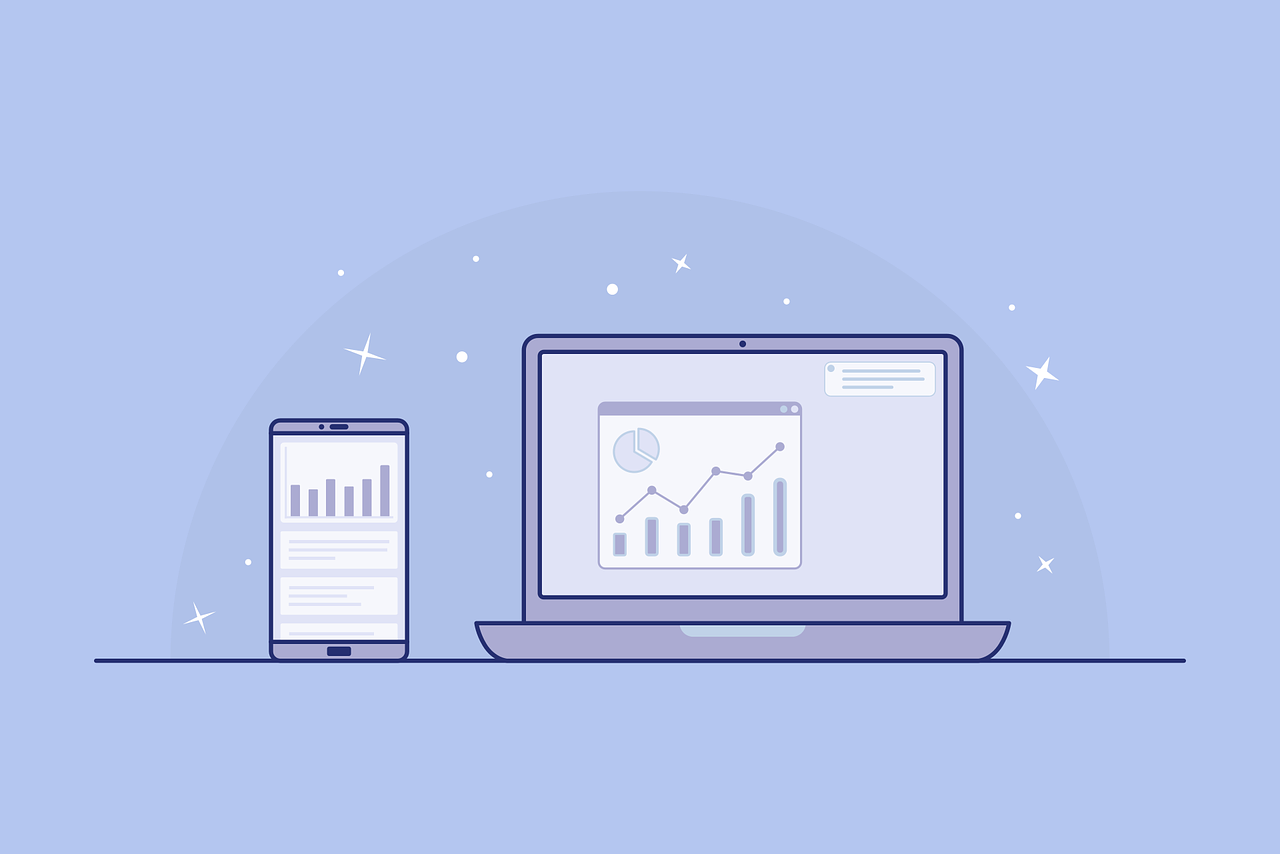
In the world of data analysis and machine learning, Principal Component Analysis (PCA) is a powerful technique that finds patterns and relationships in high-dimensional datasets.
By reducing the dimensionality of the data, PCA helps in visualizing and understanding complex datasets.
In this article, we will explore PCA in detail, from its definition and working principles to its applications and limitations.
Definition and Explanation of PCA
PCA is a dimensionality reduction technique that transforms a dataset into a new set of variables called principal components. These components are linear combinations of the original variables and are chosen in such a way that they capture the maximum variance in the data. The first principal component accounts for the largest variance, followed by the second, and so on.
Why is PCA Important?
PCA plays a crucial role in various fields, including data analysis, image processing, and machine learning. It allows us to simplify complex datasets by identifying the most significant patterns and relationships. Additionally, PCA helps in reducing noise and redundancy in the data, enabling more efficient analysis and modeling.
How does PCA work?
PCA works by performing a mathematical transformation on the data, known as basis transformation. This transformation aligns the axes of the data with the directions of maximum variance. The transformed data is represented in terms of principal components, which are obtained by calculating the eigenvectors of the covariance matrix of the original dataset.
Mathematical Background of PCA
To understand PCA fully, it is essential to grasp the underlying mathematical concepts. PCA relies on computations related to eigenvalues and eigenvectors, which are fundamental concepts in linear algebra. Eigenvalues represent the variance or importance of a particular component, while eigenvectors denote the direction or pattern associated with it.
Steps of PCA Algorithm
The PCA algorithm can be divided into several steps:
- Standardize the dataset: PCA requires the data to be standardized to ensure fair comparisons between variables.
- Calculate the covariance matrix: The covariance matrix helps in understanding the relationships between different variables.
- Compute eigenvectors and eigenvalues: Using the covariance matrix, we calculate the eigenvectors and eigenvalues.
- Sort eigenvectors: The eigenvectors are sorted based on their corresponding eigenvalues to identify the most significant components.
- Select the desired number of principal components: Determine how many principal components to retain based on the explained variance.
- Transform the data: Transform the original data into the new coordinate system defined by the principal components.
Understanding Eigenvalues and Eigenvectors
Eigenvalues and eigenvectors play a pivotal role in PCA. Eigenvalues represent the amount of variance explained by a principal component. Higher eigenvalues indicate more significant patterns or directions in the data. Eigenvectors, on the other hand, define the direction or pattern associated with each principal component. The combination of eigenvalues and eigenvectors allows us to evaluate the importance and interpretation of the principal components.
Interpreting Principal Components
After transforming the data into principal components, it is crucial to interpret the results. Each principal component represents a linear combination of the original variables. By analyzing the weights of each variable in the principal components, we can understand which variables contribute the most to a particular component. This analysis helps in identifying the underlying structure and patterns in the data.
Data Preprocessing for PCA
Before applying PCA, it is essential to preprocess the dataset. Data preprocessing involves steps such as handling missing values, outliers, and standardizing the variables. Standardizing the data is particularly crucial in PCA as it ensures that variables with larger scales do not dominate the analysis.
Choosing the Number of Principal Components
One critical consideration in PCA is choosing the number of principal components to retain. This decision depends on the amount of variance explained by each component. A commonly used approach is to set a threshold for the explained variance (e.g., 80%) and select the minimum number of components that fulfill this criterion. Alternatively, scree plots can be used to visualize the explained variance and identify the appropriate number of components.
PCA Applications
PCA finds wide applications in various domains:
- Exploratory data analysis: PCA helps in visualizing high-dimensional data by reducing it to two or three dimensions.
- Image compression: By representing images using principal components, PCA enables efficient storage and transmission.
- Feature extraction: PCA is often used to extract essential features for machine learning algorithms.
- Clustering and anomaly detection: PCA can be applied to identify clusters or detect anomalies in datasets.
Advantages and Limitations of PCA
PCA offers several advantages:
- Dimensionality reduction: PCA reduces the number of variables while preserving the most important information.
- Improved data interpretation: By transforming the data into principal components, the underlying patterns become more evident.
- Noise reduction: PCA helps in removing noise and outliers from the data.
However, PCA also has limitations:
- Linear relationships: PCA assumes linear relationships between variables, which may not be suitable for datasets with complex nonlinear patterns.
- Interpretability: While PCA provides a concise representation of the data, the interpretation of principal components may not always be straightforward.
PCA vs. Factor Analysis
PCA and factor analysis are both dimensionality reduction techniques. However, they have different objectives. While PCA aims to find the most significant components that explain the data variance, factor analysis aims to uncover latent factors or constructs that underlie the observed variables. Additionally, factor analysis allows for the possibility of measurement error, which is not considered in PCA.
Conclusion
Principal Component Analysis is a powerful tool for dimensionality reduction and data exploration. By identifying the most significant patterns in high-dimensional datasets, PCA simplifies complex data analysis tasks. Understanding the underlying mathematics and interpreting the results are crucial for applying PCA effectively. Despite its limitations, PCA remains a valuable technique in various domains and continues to contribute to the advancement of data analysis and machine learning.
FAQ
FAQ 1: What is the purpose of Principal Component Analysis?
Principal Component Analysis (PCA) aims to reduce the dimensionality of a dataset while preserving the most important information. By transforming the data into principal components, PCA helps in visualizing and understanding complex datasets.
FAQ 2: How do eigenvalues and eigenvectors relate to PCA?
Eigenvalues and eigenvectors play a crucial role in PCA. Eigenvalues represent the variance or importance of a particular component, while eigenvectors define the direction or pattern associated with it. Combining eigenvalues and eigenvectors allows us to evaluate the importance and interpretation of the principal components.
FAQ 3: How do I choose the number of principal components in PCA?
The choice of the number of principal components depends on the desired level of explained variance. A common approach is to set a threshold (e.g., 80% variance) and select the minimum number of components that fulfill this criterion. Scree plots can also provide insights into the explained variance and help identify the appropriate number of components.
FAQ 4: Can PCA handle nonlinear data?
PCA assumes linear relationships between variables, which may limit its effectiveness in dealing with datasets exhibiting complex nonlinear patterns. In such cases, other dimensionality reduction techniques or nonlinear approaches may be more suitable.
FAQ 5: What are the practical applications of PCA?
PCA finds applications in various fields, including data analysis, image processing, feature extraction, clustering, and anomaly detection. It helps in visualizing high-dimensional data, compressing images, and identifying essential features for machine learning algorithms.