Complete Guide to Principal Component Analysis
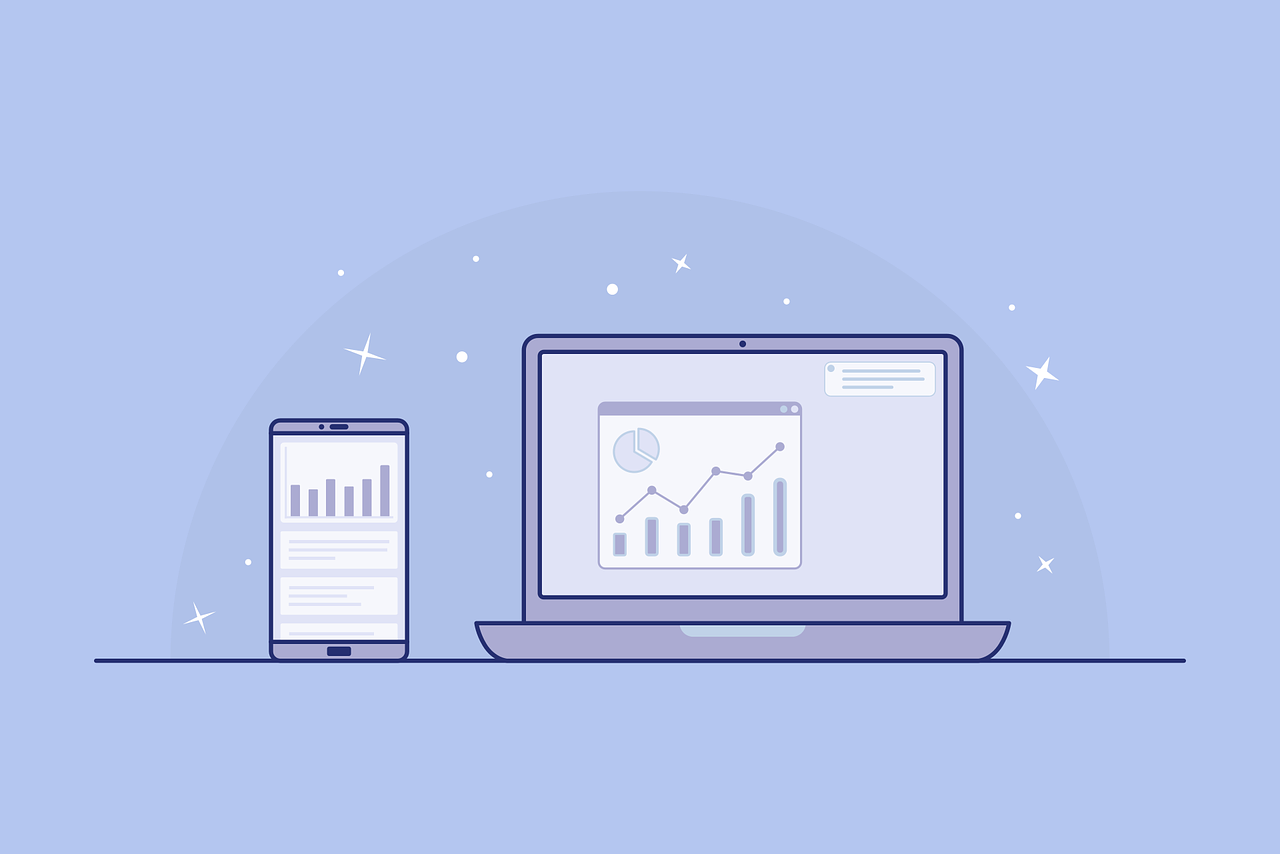
Choosing the Number of Principal Components
One critical consideration in PCA is choosing the number of principal components to retain. This decision depends on the amount of variance explained by each component. A commonly used approach is to set a threshold for the explained variance (e.g., 80%) and select the minimum number of components that fulfill this criterion. Alternatively, scree plots can be used to visualize the explained variance and identify the appropriate number of components.
PCA Applications
PCA finds wide applications in various domains:
- Exploratory data analysis: PCA helps in visualizing high-dimensional data by reducing it to two or three dimensions.
- Image compression: By representing images using principal components, PCA enables efficient storage and transmission.
- Feature extraction: PCA is often used to extract essential features for machine learning algorithms.
- Clustering and anomaly detection: PCA can be applied to identify clusters or detect anomalies in datasets.