Complete Guide to Principal Component Analysis
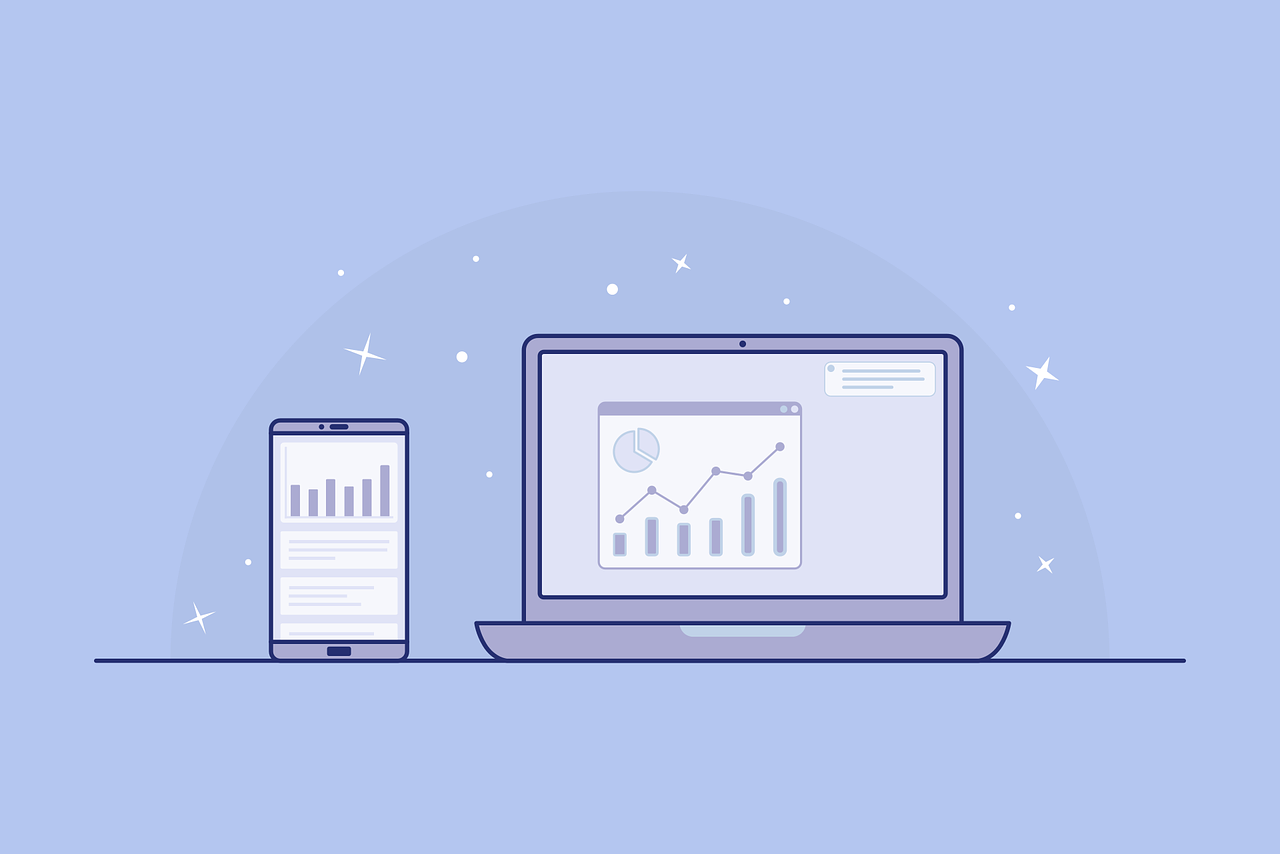
The choice of the number of principal components depends on the desired level of explained variance. A common approach is to set a threshold (e.g., 80% variance) and select the minimum number of components that fulfill this criterion. Scree plots can also provide insights into the explained variance and help identify the appropriate number of components.
FAQ 4: Can PCA handle nonlinear data?
PCA assumes linear relationships between variables, which may limit its effectiveness in dealing with datasets exhibiting complex nonlinear patterns. In such cases, other dimensionality reduction techniques or nonlinear approaches may be more suitable.
FAQ 5: What are the practical applications of PCA?
PCA finds applications in various fields, including data analysis, image processing, feature extraction, clustering, and anomaly detection. It helps in visualizing high-dimensional data, compressing images, and identifying essential features for machine learning algorithms.