Decision Trees in Machine Learning
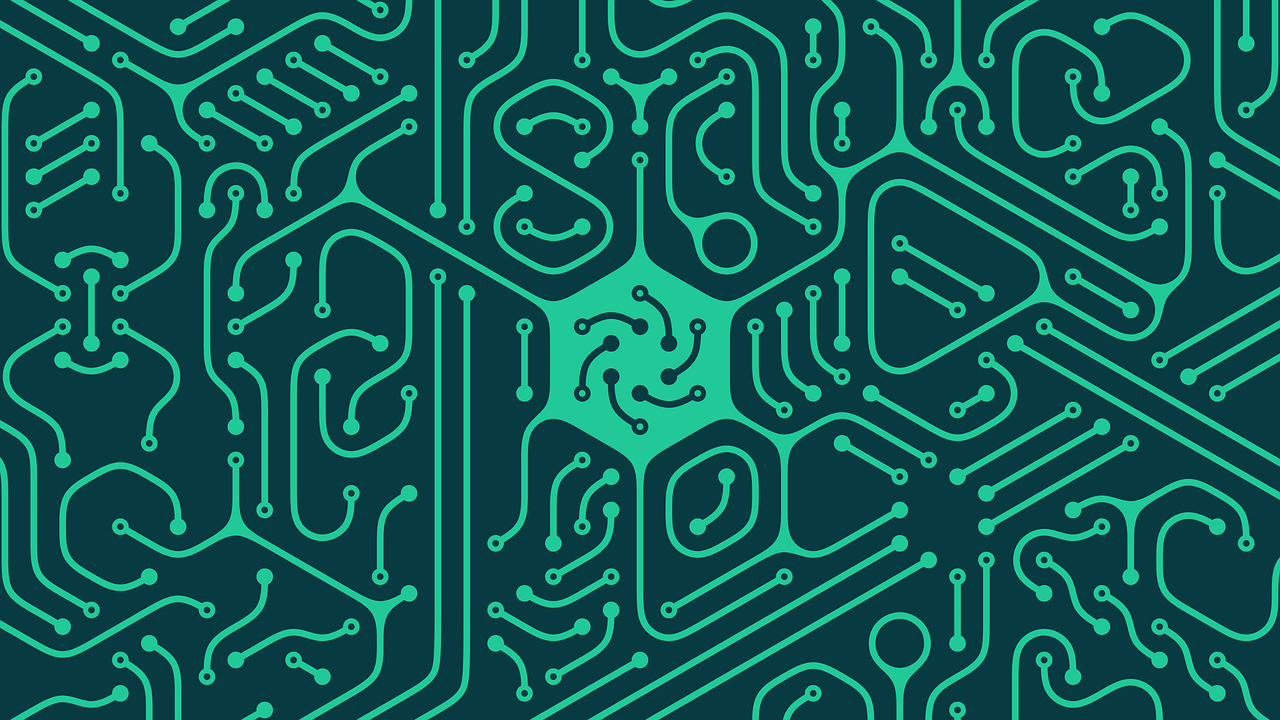
Decision trees offer several advantages that make them appealing in the field of machine learning. Firstly, they are easy to understand and interpret, making them accessible even to non-experts. Additionally, decision trees can handle both numerical and categorical data, allowing for great flexibility in different domains. They are also capable of handling missing values and outliers efficiently. Furthermore, decision trees can be visualized, providing clear insights and explanations for decision-making.
Disadvantages of Decision Trees
Despite their many advantages, decision trees also have their limitations. One of the drawbacks is their tendency to overfit the training data, resulting in suboptimal performance on new, unseen data. Decision trees are also susceptible to small changes in the dataset, potentially leading to different tree structures. Additionally, complex decision trees can become difficult to comprehend and prone to inducing biased or inaccurate predictions.